July 15, 2019
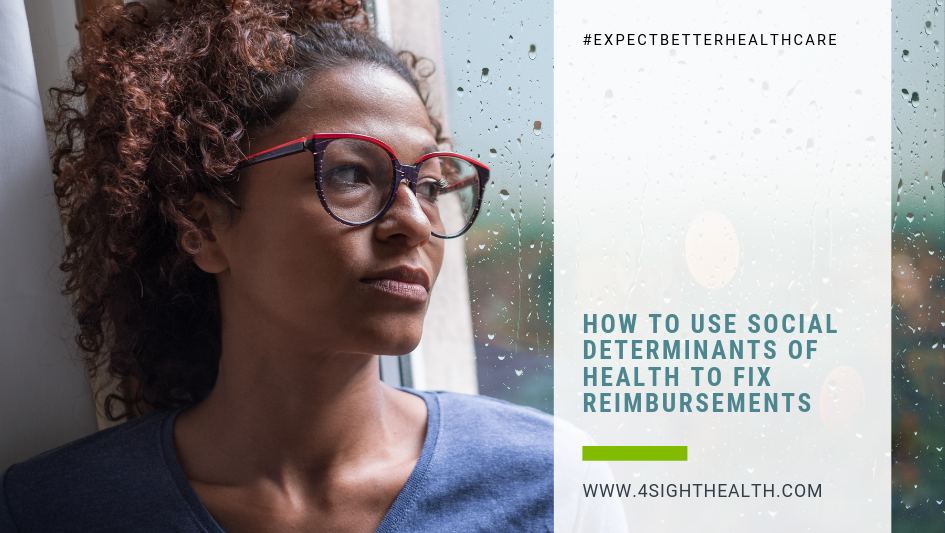
How to Use Social Determinants of Health to Fix Reimbursements
Healthcare providers can no longer ignore the Social Determinants of Health (SDH) in the exam room. Even John Snow considered community assessment factors to identify the source of the cholera epidemic in 1854. Positive health outcomes are reliant on managing SDH, so when will states begin to incorporate SDH into their payment reimbursement models?
Using big data analytics to measure SDH factors will improve healthcare outcomes at lower costs. States’ ballooning Medicaid budgets are signing more risk-based contracts with health systems and managed care organizations. Instead, financial incentives should flip from volume to value and states should invest in SDH innovations.
Providers know that the diabetic patient who has a support system and access to healthy food is more likely to lower their A1c, than a patient who lives alone in a food desert. We now know that a person’s zip code (“ZNA”) affects their health more than their genetic code (DNA).[1] To improve health outcomes, states need to address this elephant in the room.
It is the government’s and society’s duty to improve Medicaid programs.Over 35% of the U.S. population (more than 113 million people) depend on publicly funded programs. If SDH continues to be ignored, poor health outcomes will perpetuate and costs will rise.
There is a better way. States can learn from the examples of Massachusetts and Minnesota who have used big data analytics to transform their Medicaid programs. Massachusetts was the first to incorporate “unstable housing” and “neighborhood stress score” factors into the risk-adjusted capitated premium for Medicaid ACO enrollees. Dr. Arlene Ash from the University of Massachusetts said, “the risk-based payment model for MCOs and ACOs that we built for MassHealth now adjusts not only for age, sex and medical diagnoses, but also for social risks (including disability, housing problems, and neighborhood indicators of economic stress).”[2] None of these factors come from traditional sources of data, such as medial claims and EMRs.
Similar to Massachusetts, Minnesota is also tracking a wide range of non-medical data to assess their impact on health outcomes. Here are five key factors:
- Homelessness
- Immigration status
- Primary language
- Number of children in the household
- Involvement of child protective services
Since 2018, Minnesota’s Medicaid program has worked on ways to incorporate the results into future payment models and interventions. Without big data analytics, the states cannot measure the results of their payment models accurately.
SDH should factor into how healthcare providers on the frontline get paid. By turning the payment model upside down to value, innovative payment models that take SDH into account upfront will give providers room to invest in grant-funded or unfunded programs to address health disparities. It is time for states and providers to succeed with the highest risk, most vulnerable populations by providing the right care at the right time in the right place. For more information on this topic, please read the full Market Corner Commentary here.
SOURCES
[1] https://www.salon.com/2016/11/12/how-zip-codes-have-an-impact-on-health/
[2] http://www.themedicalcareblog.com/risk-adjustment-interview-with-arlene-ash/